Which skirt is currently particularly popular? Should the dress remain on sale? How did the sweater sell last month? Product managers in the fashion industry ask themselves these and many other questions every day. At bonprix, the ‘Learning Collection’ forecasting system has been helping them find answers for some time now. Jessica Külper, Head of Strategy and Innovation Management, explains why the company uses modern technology to help with product selection and how it works.
Ms. Külper, how have you planned your product range before the use of AI?
Jessica Külper: The product range we have created so far is based on the experience and knowledge of our product managers, for example with regard to the needs and wishes of our customers. Competitor analyses and current trends are also factored into[JP1] into the planning process.
For some time now, the ‘Learning Collection’ forecasting tool has been influencing your assortment policy. How is it used?
‘Learning Collection' is a new forecasting system developed in-house at bonprix that uses artificial intelligence to make reliable predictions about demand and purchases. The system evaluates new product ideas from bonprix and forecasts which of the articles are likely to sell well and which are likely to be in low demand. Based on this analysis, our employees can plan the product range even better and thus align it even more closely with the wishes and needs of our customers.
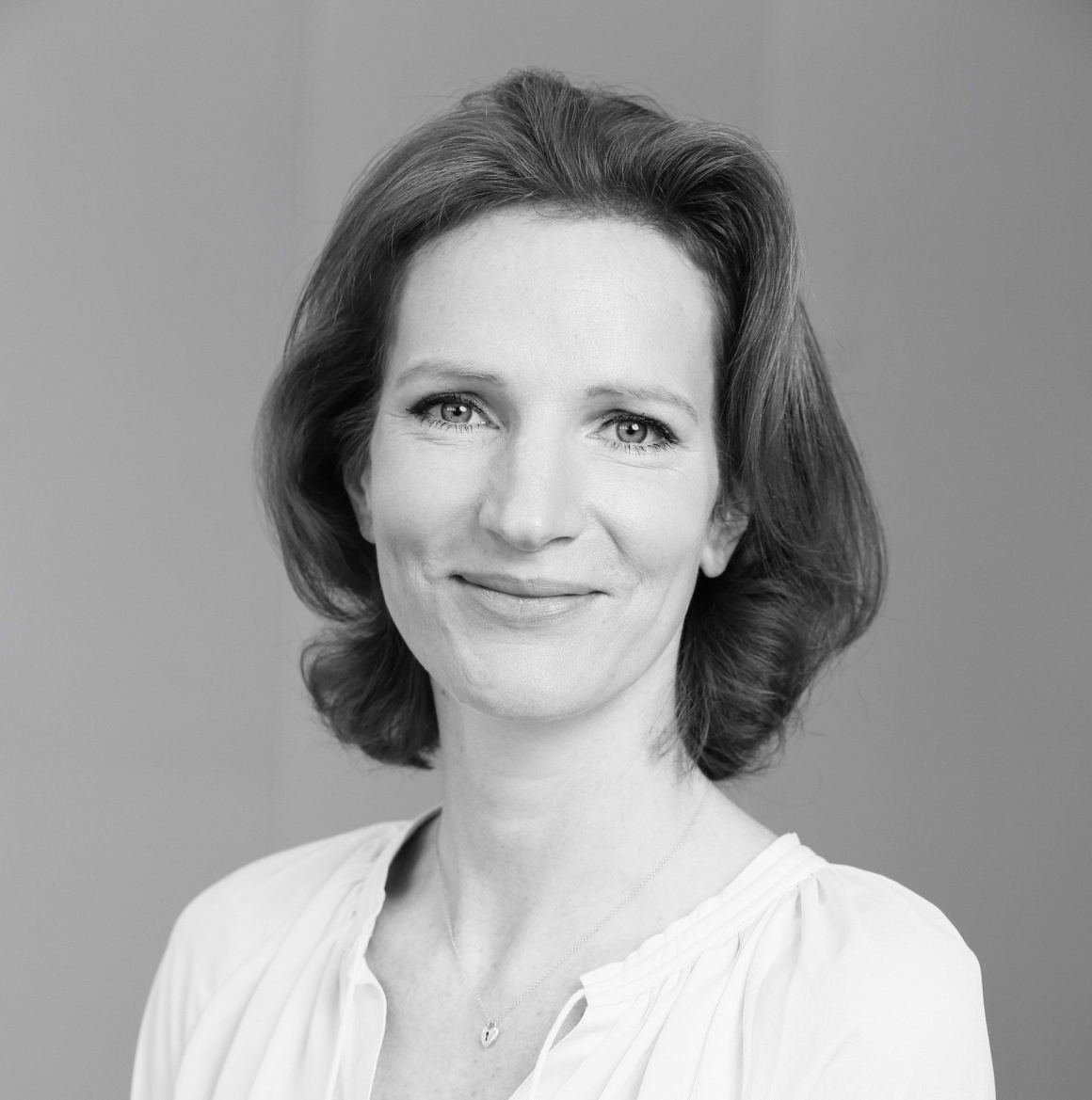
What technology is behind this?
The tool for this forecasting system, the ‘article-specific success analysis’ (ASA), was developed by bonprix together with AI experts from the Otto Group Data Lab. Behind the forecasting system is a machine learning algorithm that continuously optimizes itself on the basis of newly acquired information so that the forecasts become increasingly precise.
To be more specific, the model is continuously fed with historical data and trained to recognize regularities in the abundance of data and to deliver forecasts. All previous bonprix articles – including article attributes and sales figures – are fed into an algorithm and the system recognizes correlations and patterns in the data. We regularly enter collections and forecast which articles will be in high demand (top articles) and which will not sell well (flop articles). Products that are predicted to sell poorly are not included in the product range; other articles are optimized, for example by adjusting the color.
But what's trendy today may be passé tomorrow, right?
We regularly check how accurate the model's forecast is and how it corresponds to actual sales figures. If the forecast is consistently good over several months, it will most likely be reliable in the future. And the more information that is fed into the algorithm over the months and years, the better the forecasts can become over time.
Why are such tools needed these days?
Artificial intelligence offers great potential to improve forecasts and make processes more efficient. In the end, humans still have the final say. But AI can provide valuable input through its ability to take into account a wide variety of influencing factors in order to develop the truly best styles for customers.
Was it hard to get started? How did you approach the implementation?
We were curious about the extent to which artificial intelligence could meaningfully help us plan our assortments in an even more efficient and customer-oriented way. To find out, we started with various test runs. Based on all product data from 2016 to 2018, we had the algorithm predict the year 2019. This test was carried out at the end of 2019, so we were able to assess the forecast quality directly – and the result impressed us. The forecast was so good that we subsequently started the implementation. The ‘Learning Collection’ tool was launched in summer 2020 and has been used at bonprix every month since the creation of the January 2021 collection.
What have you learned about customer preferences and behavior since then that you didn't know before?
From the enormous wealth of data, the algorithm can not only rank item demand based on individual product attributes, but also predict which combinations of different attributes are most promising. This has given us some new insights into what our customers want. One example: We found out that overalls with three-quarter-length pant legs are much more likely to be purchased by our customers than overalls with long or very short legs.
How has the product manager's job changed since then?
The processes for inspiration, assortment and collection creation have not changed noticeably, as the insights from the AI logarithm are available at the push of a button, so to speak, to be incorporated into the final assortment design. Thus, the forecasts are a useful addition to personal experience, knowledge of fashion trends and expertise – because even with the support of AI data, the expertise and gut feeling of our product managers remain essential when deciding on articles and collections.