When it comes to using and analyzing relevant data, brick-and-mortar retail lags behind its online competition. While e-commerce retailers use detailed data from web analytics, CRM, and Google searches to analyze user behavior and generate personalized offers, the majority of conventional retailers and brands is still a long way away from utilizing this type of information.
If retailers or brands in the analog world want to determine the best location for opening a new retail outlet, find out how to optimize their product assortment or how to target their limited marketing resources, many of them still rely exclusively on static data structures or the implicit knowledge of their employees. Despite the broad availability of digital geodata, offline retail often still relies on a limited amount of information and gut feelings to set strategic directions.
In order for brick-and-mortar retail to get on board with data-driven decisions, there is now a scalable approach that forecasts sales for different points of sale, segments outlets based on their sales potential and product-market fit, and optimizes marketing efforts per store – and all based on different forms of digital data. This approach, developed by TD Reply and called "Advanced Outlet Analytics", can be applied in many industry sectors. Examples include food and beverage retail, fashion or the automotive sector.
Earned data: hidden digital data trove for retailers
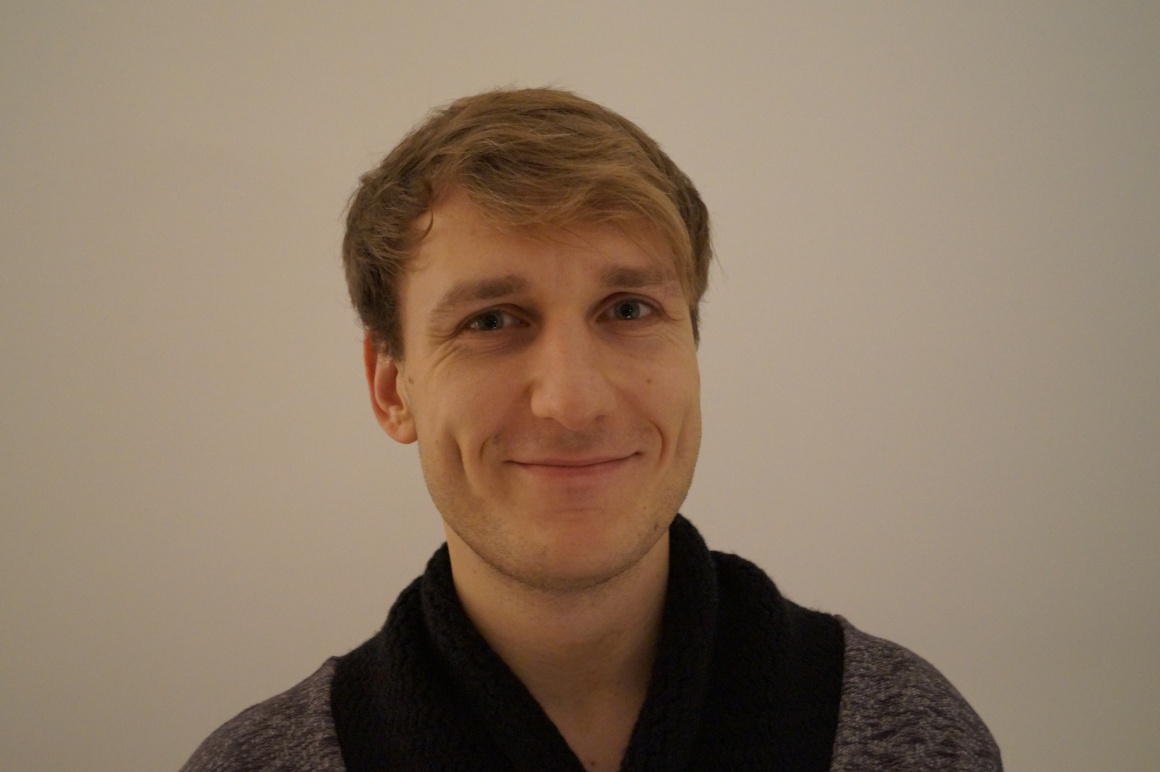
B2B sales is challenged with targeting those outlets that are likely to result in the best sales prospects for the product in question. B2C retailers face a similar problem. They need to consider issues like, “Which categories and products have top selling potential based on the store, and how much inventory should be carried in these respective instances?” Answers to these questions can be obtained from information such as a company’s proprietary data (owned data) like first-hand sell-in or sell-out data. Brands without access to owned data or where data quality is insufficient might also consider purchasing sales data via digital POS providers (so-called paid data).
That being said, even if product sales data is available only a few companies have a clear idea as to what external factors influence sales performance and what the most effective levers are for maximizing success. Often going unnoticed by offline retail, answers to these questions can be found online.
Web services like Google Maps, Facebook or Flickr offer large amounts of geo-specific data with high information value. Brick-and-mortar retailers can adopt the view of the end consumer to find the most relevant information for their respective line of business. As an example, consumers search online to find out business hours (Google), user rankings and ratings (Google, Tripadvisor, Foursquare), store-specific offers and services (Yelp), directions (Google Maps) or relevant events and opportunities (Facebook). This behavior generates geographical data that can be personalized for each outlet and simultaneously scaled for the entire market.
Just by utilizing data from geographic services like Google Places or Open Street Map alone, retailers can get detailed insights into the surrounding area of a respective outlet. This includes information such as store density, relevant venues such as concert halls or schools, or proximity to train stations.
Integrating this data with proprietary numerical data is a critical and so far missing link that allows detailed evaluations and assessments of the relevant target market, corresponding sales potential and the most effective marketing efforts per outlet.
Forecasting sales and reducing out-of-stocks
One use case for the intelligent application of online data is improved sales forecasting, which can achieve over 90 percent accuracy in food and beverage retail for example. In addition to the sell-out data per outlet, data also includes information about local events obtained courtesy of Facebook, Google data on regional search interest and current weather data.
Based on these more accurate data-driven forecasts, the number of deliveries can be reduced by up to 60 percent, while the risk of out-of-stocks can be reduced at the same time. It also enables better scheduling of sales representative visits, which significantly increases cost efficiency.
Segmented market development – based on digital data
Another approach to using external online data is data-driven segmentation of outlets, which is relevant to both retailers and brands as it pertains to product assortment and marketing optimization. Information about restaurants, bars and hotels in the surrounding area (retrieved from Trip Advisor for example), the potential for high frequency of a respective location (via information from Google Places) or destination attractiveness of a vicinity (based on the number of photos uploaded on social media for instance) can allow predictions of potential sales volumes and product-market fit to prioritize outlets and assign them to specific brands and products.
If these segmentations are linked with information on marketing efforts and sales data, businesses are able to specifically prioritize the spots where corresponding activities pay off – including in-store promotions, sales promotions or a change in the product mix.
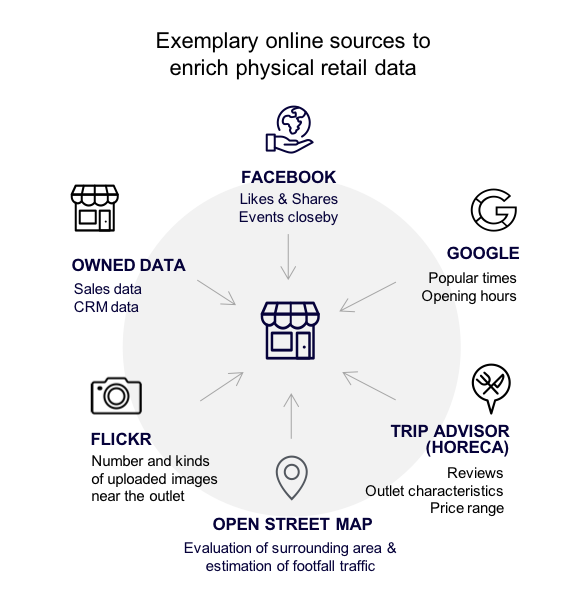
Turning big data into smart data
Taking advantage of the latest technologies such as machine learning and artificial intelligence also plays a central role in making the aforementioned approaches scalable and boosting continuous improvement. It is crucial that the models update dynamically and respond to changes in data and environmental factors.
Having said that, new technologies are not a silver bullet for business operations. New data and technologies are only an effective tool if they are linked to concrete questions and the implicit industry knowledge mentioned earlier. The iterative review of the generated results in the field and the subsequent optimization of the created models are very important in this setting.
"Advanced Outlet Analytics" is not a purely statistical approach that is being developed surreptitiously. It actually requires continuous adjustment to market reality and the existing knowledge of local sales and store managers. "Data science" and "machine learning" are the buzzwords of the moment, but either one can only be fully effective in communication with the entrepreneurial base.